Artificial intelligence (AI) is a fascinating and constantly evolving field, but it is complex and fraught with unexpected events. To succeed in your AI projects, it is crucial to understand and master several fundamental aspects. Here is an in-depth exploration of three key elements that should not be overlooked to ensure the success of your AI initiatives.
Developing your own AI is not just a matter of technology or technical expertise. It involves a holistic understanding of processes, resources, performance measures, and the adaptability needed in the face of uncertainty. It requires a strategic approach, careful planning and agile execution.
- Fragmentation of work
Data dependence: Data is the foundation of any AI project. Their quality, accessibility and relevance are decisive for the success of the project.
Building AI is inseparable from data processing and analysis. However, data management is often fragmented and relies heavily on human input.
Data collection and processing involves human interactions, which may be uneven and discontinuous. This intermittency requires flexible planning and dynamic team management.
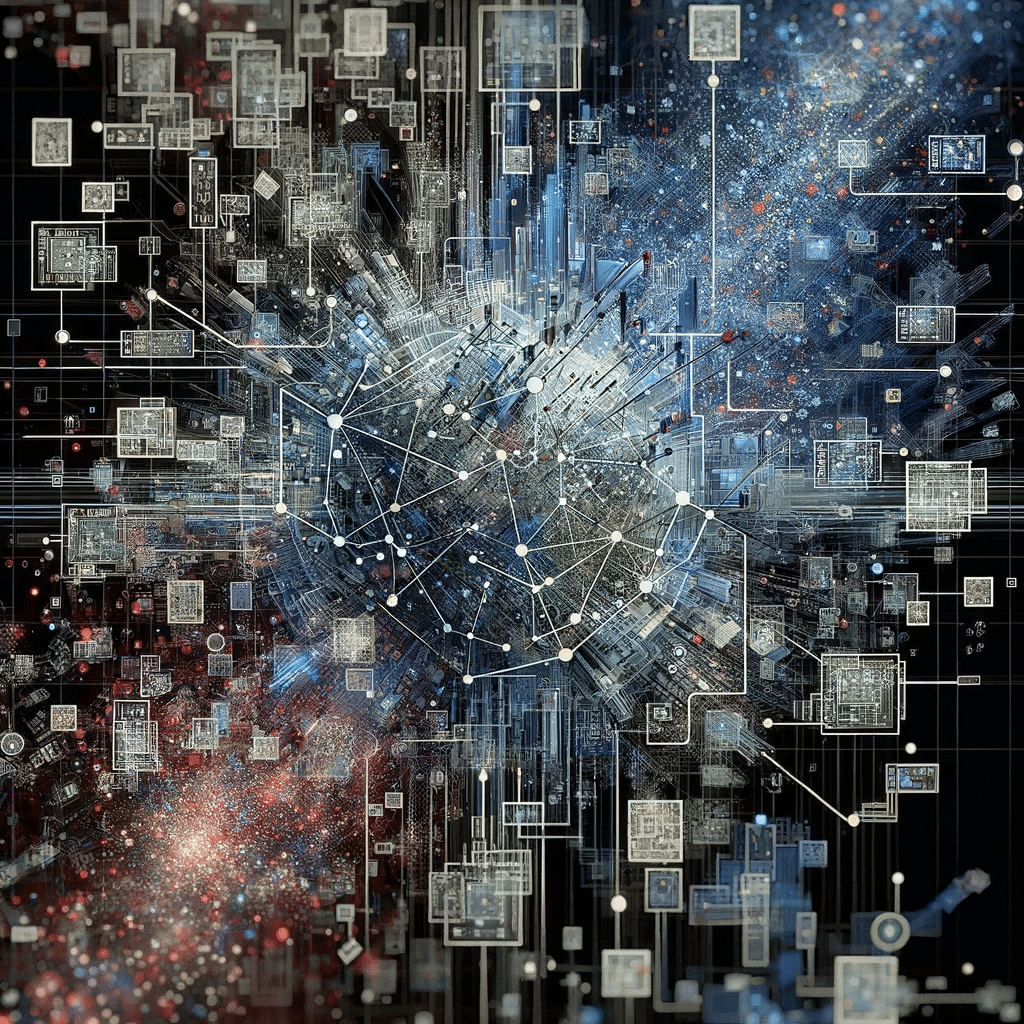
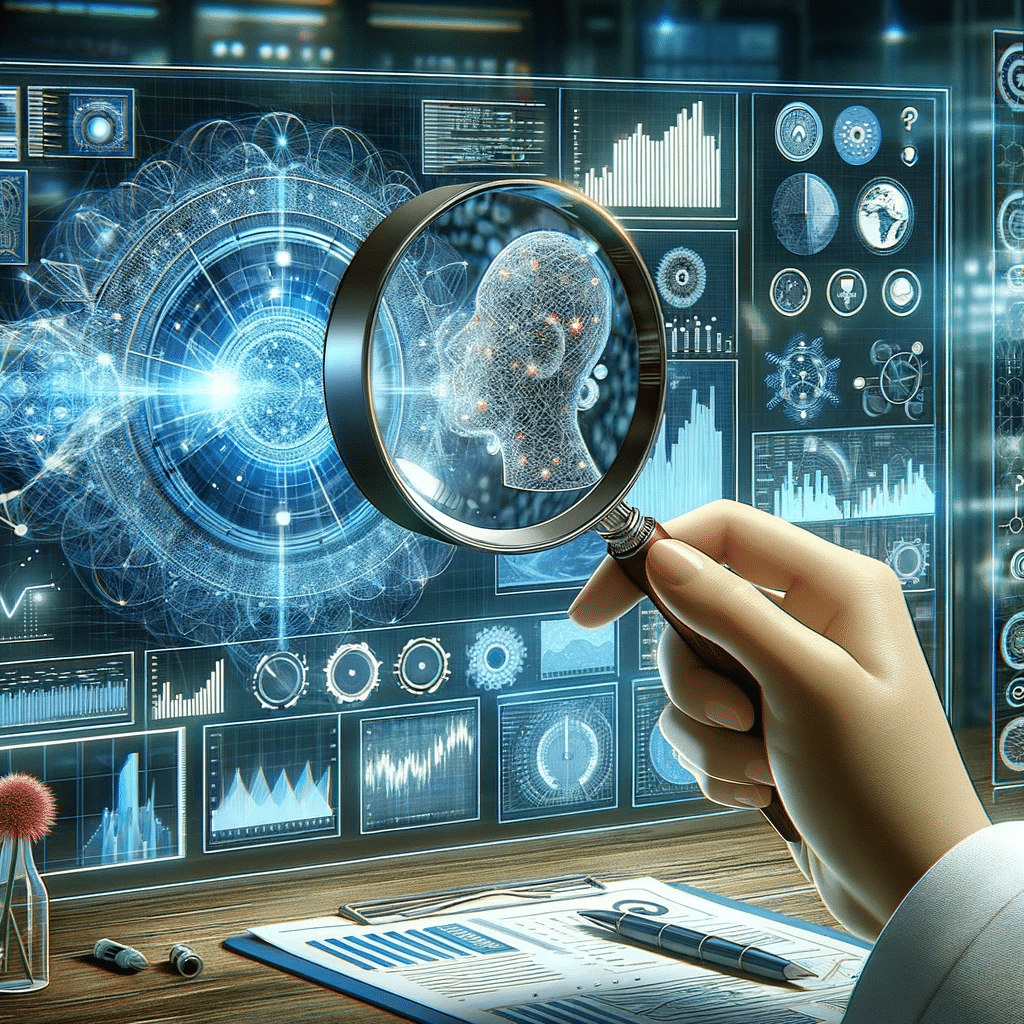
- The importance of measurement
Measurement is essential for tracking progress and assessing the actual performance of AI.
Definition of indicators: It is crucial to define clear and measurable performance indicators from the start of the project. This allows progress to be monitored objectively.
Continuous measurement: Constantly monitoring these metrics helps adjust strategies in real time and ensure the project stays on track.
- The uncertainty
Uncertainty is an inevitable aspect of AI projects.
- Need for short iterations: Faced with this uncertainty, it is advisable to adopt short iteration cycles. This allows you to test, learn and adapt quickly. Furthermore, it is the best way to manage the risk of failure (in addition to being the best way to reduce it).
- Abandoning the traditional project model: It is important to break away from the idea of a massive and entirely plannable project, for which we spend months writing specifications, two years implementing it, and an eternity to cry because the user doesn't want it.
Taking an agile and flexible approach is more effective.
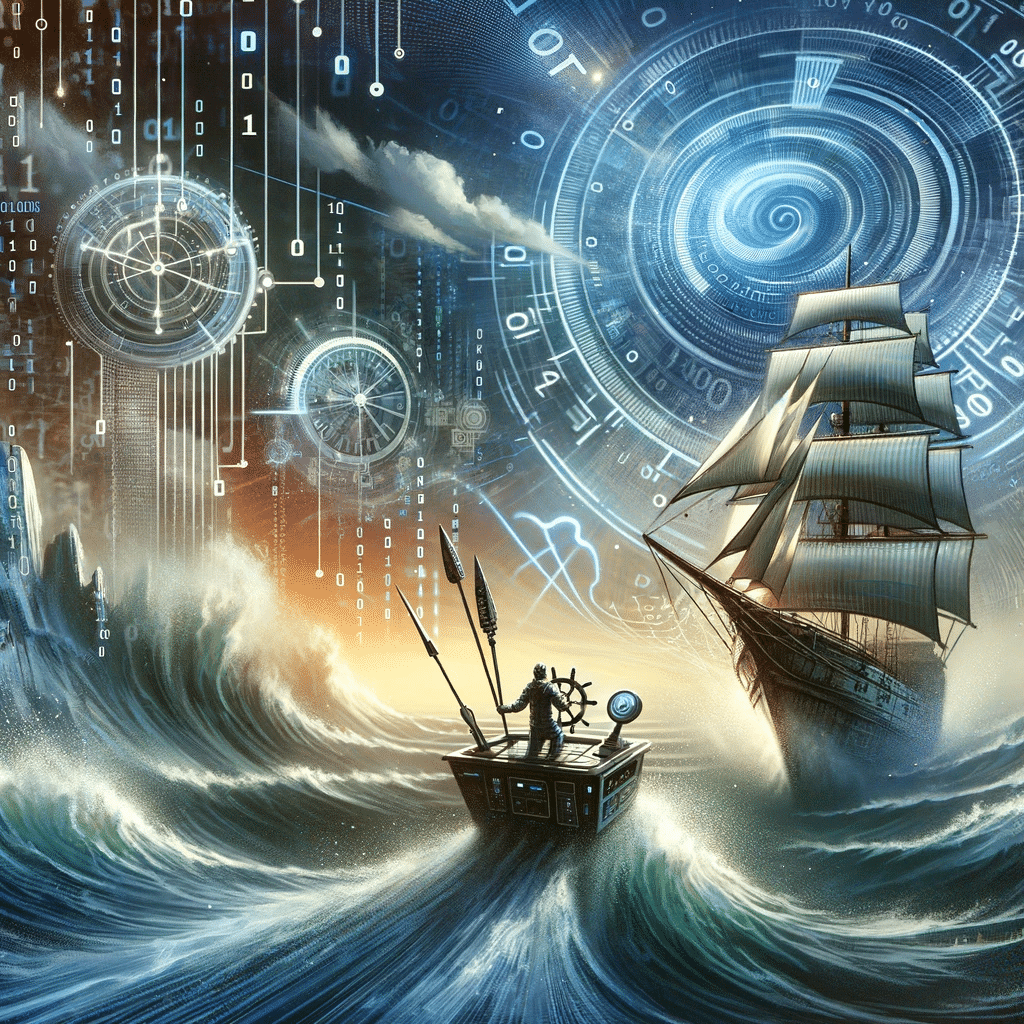
To succeed in AI projects, it is essential to take into account the fragmented nature of the work, the importance of measuring performance from the start, and knowing how to navigate the uncertainty inherent in this field. This involves agile human resources management, a clear definition of success indicators and an acceptance of iteration as a working method. By embracing these principles, AI projects can not only achieve their goals, but also pave the way for unexpected innovations.